Category: Monday Magazine
Though we are an aggregator blog (providing links to content elsewhere) on all other days, on Mondays we have only original writing by our editors and guest columnists. Each of us writes on any subject we wish, and the length of articles generally varies between 1000 and 2500 words. Our writers are free to express their own opinions and we do not censor them in any way. Sometimes we agree with them and sometimes we don’t.Below you will find links to all our past Monday columns, in alphabetical order by last name of the author. Within each columnist’s listing, the entries are mostly in reverse-chronological order (most recent first).
The atom bomb and the two cultures: I.I. Rabi on the sciences and the humanities
by Jeroen Bouterse
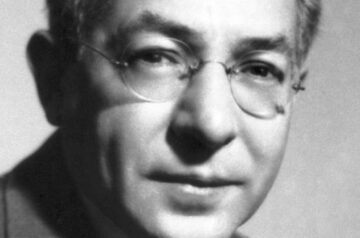
Several years before C.P. Snow gave his famous lecture on the two cultures, the American physicist I.I. Rabi wrote about the problem of the disunity between the sciences and the humanities. “How can we hope”, he asked, “to obtain wisdom, the wisdom which is meaningful in our own time? We certainly cannot attain it as long as the two great branches of human knowledge, the sciences and the humanities, remain separate and even warring disciplines.”[1]
Rabi had been interested in science since his teenage years, and grown up to be a Nobel-prize winning physicist. He had also been an important player in the Allied technological effort during World War II, as associate director of the ‘Rad Lab’: the radiation laboratory at MIT that developed radar technology. The success of Rad Lab, Rabi later reflected, had not been a result of a great amount of theoretical knowledge, but of the energy, vitality, and self-confidence of its participants.[2] In general, Rabi’s views on science and technology were somewhat Baconian: science should be open to the unexpected, rather than insisting on staying in the orbit of the familiar.[3]
‘A moralist instead of a physicist’
In Rabi’s accounts of his time leading Rad Lab, he would also emphasize the way in which he insisted on being let in on military information. “We are not your technicians”, he quoted himself, adding: “a military man who wants the help of scientists and tells them half a story is like a man who goes to a doctor and conceals half the symptoms.”[4] Indeed, the key to understanding Rabi’s worries about the two cultures – he would go on to embrace Snow’s term – is his view of the role science ought to play in public life. Scientists should not just be external consultants,[5] delivering inventions or discoveries on demand or listing the options available to the non-specialist.[6] In some stronger sense, they should be involved in directing policy decisions. Read more »
Fabula rasa
by Rafaël Newman
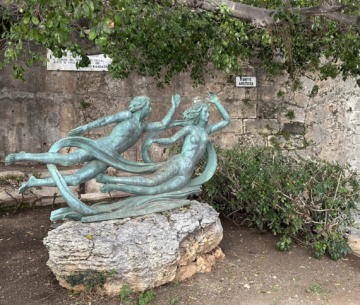
The initial syllable of the English word “island”—or rather, just its very first vowel—is descended from the Proto-Germanic *awjo, meaning “an area on the water.” The element “land” was subsequently added to differentiate the word from other inflections of the Proto-Indo-European root for water, *akwa-. Our “island” is thus cognate with its German counterpart Eiland, although these days German speakers probably prefer Insel, derived from the Latin insula, which refers less ambiguously to a land mass entirely surrounded by water and not, as Eiland can, to a territory simply suffused with water.
The “s” in our “island,” however, was inserted much later, by a process of classicizing back-formation, to associate it with its etymologically unrelated synonym “isle”: as if to establish a linguistic lineage, to give island’s humble Germanic form a noble Roman pedigree. To link it, as it were, with another, greater, more patrician history.
The desire to link islands up, to settle them down, to connect them with the mainland, is an old one. Our continents, after all, are thought to have derived from the drifting apart of an original, unitary Pangea, and perhaps we have been yearning ever since for a return to that felicitous Ur-conglomerate. “No man is an island” (or rather, “iland”), wrote John Donne in 1624. Fast forward to the Confederation Bridge, or “fixed link” that unites Prince Edward Island with New Brunswick, inaugurated in 1997, and you can trace the modern history of a venerable conviction. Read more »
Perceptions
The Utopian Impulse (Part I)
by Angela Starita
New York magazine published a story about two sisters who decide to leave civilization and try surviving in the outdoors of Colorado. One has a 14-year-old son, and she brings him too. In fact, much of her motivation appears to be protecting him from dangers she perceives, existing ones and more she believes to be just on the horizon. The writer doesn’t know many of the specifics of those fears, but from text messages, she gleans that the mother, Rebecca Vance, believed that the world was on the verge of collapse and wanted to escape the ensuing survivalist brutality. Instead, the three died of malnutrition and hypothermia probably within two months of going “off grid.” In short, in trying to outrun anticipated violence, they instead faced a grueling, attenuated death.
People quoted in the article attest to the sisters’ clear lack of familiarity with the backwoods camping let alone how to build a shelter, gather or grow sufficient food, generate heat, and all the other essentials to assure long-term survival. That aside, though, the sisters were acting out a typical response to their fear of the encroaching demands of the modern world. In their own panicked, inept way, the sisters were part of a long tradition of runaways looking to escape or reconfigure the rules of society as they understood them. It’s a familiar list—New Harmony, Oneida, the Shakers, even Jonestown—born of a familiar impulse: to start over and this time get it right. Read more »
Tempus Fuckit
by Akim Reinahrdt
Time slips
past us, fast flow,
like a river rushing over gray stones
Time drips
slower than slow
like thick sap hanging from pine cones
I’m not sure time is real. I mean, things happen. Entropy and whatnot. But I don’t know if I accept that measuring the pace of happenings is anything more than a construct.
Don’t get me wrong. I know the world is round, or a close approximation thereof. I’m down with the science. But physicists, as a group, aren’t united on what time is. Something about time being “measured and malleable in relativity while assumed as background (and not an observable) in quantum mechanics.”
So while we experience it as real, it may not be “fundamentally real.”
And that’s kinda how it feels to me.
I remember my 6th grade English teacher, Mrs. Newman (Ms. was not to her liking), telling us that the older you get, the faster time goes by. I’m not sure why, but that idea immediately clung to me. Though I was only 11 years old, or perhaps in part because of it, I got what she was saying. And I believed her. After all, she had lived four or five or six times (who could tell) as long as I had. So even though what she was describing sounded like a cliché passed on from generation to generation, I assumed her own experiences had borne it out. During the four and a half decades since, I have always remembered her words and noticed that, in a general sense, she was absolutely correct. Back then, a summer was endless. Now, the years roll on like a spare tire picking up speed down a hill.
But that is a historical observation I make as I look back. My present, like everyone else’s, stretches and squeezes like an accordion. Read more »
Catspeak
by Brooks Riley
Activism as Art (Inaugural Rosemary Bechler Memorial Lecture)
by Gus Mitchell
This article is from a presentation made for the Rosemary Bechler Inaugural Memorial Lecture, an event organised by DIEM25, of which Rosemary was a founder member. It took place at the Marylebone Theatre in London on January 21st 2024.
That music you just heard is a recording of the Aka People, recorded in the forests of the Central African Republic. The musicologist who collected it gave it the simple title: “Women Gathering Mushrooms.” I like that title because I think its literalness reveals something important. I don’t know if the Aka conceive of it as “art” in the same way that now, sitting in a theatre in London in 2024, we conceive of it. Undoubtedly though it is art in the truest sense.
Of course, it is very difficult––it is impossible––to avoid speaking in generalities when you’re using a word as general, as vast, as art. There are as many arts as there are artists. Avoiding abstraction is impossible. But that’s also part of what I’m going to try to say here. That is––that most of the ways we tend to think about art today are abstract. Too much so, I think. I think that art might in fact mean more than we currently allow it to. It might be more than we currently allow it to be. Read more »
Yalom’s Gift
by Marie Snyder
I recently binge-watched all of Group, a show inspired by the Irvin Yalom novel, The Schopenhauer Cure. So I revisited Yalom’s non-fiction to see how closely the series aligns to his actual practices.
The Gift of Therapy is a fascinating read from 2017 in which Yalom dives openly into his existential psychotherapy practice, explaining the four givens that affect how we think, feel and act that need to be explored at depth: death, isolation, meaning of life, and freedom (xvii). In the introduction, he jumps right into death denial revealed through a belief in personal specialness (xiii). Our current culture of selfies is likely rife with this! An existential perspective is best for clients who despair from “a confrontation with harsh facts of the human condition” (xvi). We didn’t see much of this type of discussion in the show. In fact, the therapist didn’t talk much at all beyond reminding the group to be honest and forthcoming. Read more »
Monday Photo
On the Road: On Shaky Ground
by Bill Murray
The Balkans isn’t everybody’s first choice for summer holiday, but that’s where we’re headed this year. First we’re flying to Chișinău, while we still can, and I don’t mean to be flip. Forgive my wavering confidence in Western guarantors of freedom, democracy and territorial integrity.
My idea then is to press south from the Moldovan capital through Romania and Bulgaria. Later we’ll head to Skopje, Lake Ohrid, Pristina and Tiranë. No Disneyland for us this summer. We’re going to Plovdiv.
Where my wife comes from in Nordic Europe, thuggery isn’t street crime and graft. Up there, thuggery plays out against dramatic backdrops, with a sense of the cinematic. It’s oligarch families flying drones through the Arctic, or it’s cynical manipulation of human lives, Russians importing Somali “asylum seekers,” then renting them bicycles to pedal off toward the Finnish border.
In the Balkans it’s not like that. Down there, we’re led to believe unkempt rogues and opaque political intrigue are common as London fog. In the Balkans, they say, thuggery is bona fide. Local. Home grown. And scoffing at the law starts with the leader. Read more »
On the 12/8 Path with Charlie Keil
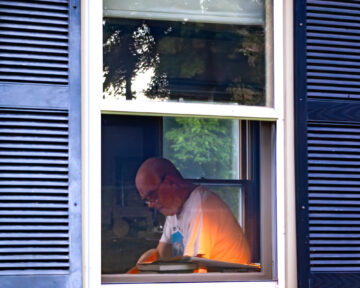
I knew about Charlie Keil somewhat before I met him and long before we began collaborating on various projects. In 1966 he published Urban Blues, which was a study of such singers as Bobby Blue Bland and B.B. King, blues musicians who wore sharp suits and performed in urban venues with electrified instruments. The book received wide acclaim, both in intellectual circles and in, of all places, the rock and roll press. And why not? After all, much of rock and roll was based on the blues. This lively and erudite book, published by an prestigious university press, University of Chicago, gave legitimacy to the work of critics publishing in Rolling Stone, the Village Voice, and Crawdaddy.
I don’t know just when of how I came to read it, but it electrified me. For not only did Keil write about the music, he wrote about the musicians and the communities in which they lived and performed. After all Keil was an ethnomusicologist, and it probably said so somewhere on or in the book. If so, it didn’t register with me. And, like many who read the book, I assumed that Keil was black. I mean, how could a white man know so much about black life and write so sympathetically about it? No, this Charlie Keil fellow had to be black.
While I might has bought the book soon after it was published, I don’t actually know that. But I’m sure I bought it sometime before the fall of 1973, when I went off to graduate school, because I remember talking with my Baltimore friends about the book, who were as taken with it as I was.
When I shuffled off to Buffalo – yeah, the Devil made me do it – I didn’t know that Charlie Keil was on the faculty there. I went off to the English Department at UB (State University of New York at Buffalo) while he was in American Studies. But I also hung out in the improvisation workshop run by Frank Foster in the music department. Though I never verified this, I had the impression that some of the people in the workshop were music students at Buffalo, while others were young local musicians who showed up to learn from a man who’d played with Count Basie, Sarah Vaughan, Elvin Jones and who knows whom else. Frank was one of the masters, and we were there to learn from him. Read more »
Monday, January 22, 2024
Ignorance and Blame During the Recent Alien Invasion
by Tim Sommers
Does ignorance always excuse someone’s actions no matter how much harm they cause? If so, doesn’t that imply that the less I know about the problems of the world the less I can be blamed for them? Paradoxically, can I be a better person by remaining more ignorant? Or can I be morally culpable despite being factually wrong or ignorant about something? Either possibility seems unsettling.
Suppose malevolent aliens philosophically dedicated to chaos and destruction invade the Earth just for the sake of creating havoc and killing as many humans as they can before they move on to the next planet. As part of the struggle for survival, governments create and distribute an “alien repulsive device” (ARP). About the size and shape of a wristwatch, the ARP emits a frequency of electromagnetic radiation that discourages, but does not totally prevent, alien attacks. During the first ten months after the ARP device is distributed the authorities estimate that 200,000 lives are saved in the US alone, and a million and a half hospitalizations due to alien injuries are prevented.
Nonetheless, there is a group of people who oppose the use of ARPs. Some argue it is just a way for governments to track your movements. Others argue that the device does more harm to the wearer than is justified given that the risk of an alien assault is quite low – and the results are usually not that bad. Others deny the existence of the aliens altogether.
As a result of these views, ARP/alien-deniers spread the word to the world. Do not wear ARPs! They blog, they podcase, they protest, they try to prevent the government from handing them out by threatening or harassing public health officials. Some harass total strangers in public settings for wearing ARPs. Doctors and demographers estimate that a third of a million additional people die as a result of the alien-denialist movement, people who otherwise could have been saved.
Since this is a hypothetical, let’s just stipulate that no reasonable person could deny that there was an alien invasion, that on-balance ARPs saved lives and seriously mitigated harms, and that ARPs cannot be used as trackers. Given this, are deniers morally culpable for any of these excessive deaths? Read more »
Big Money Guaranteed!
by Jonathan Kujawa
The State of Georgia has a lottery guaranteed to turn an enterprising 3QD reader into a millionaire [1].
Playing the lottery can be fun. It is a tradition for Anne and me to buy lottery tickets when stopping for gas on long road trips. Imagining what you’d do with our new wealth is a fine way to pass the long hours it takes to cross the panhandle of Texas. Occasionally, we win back the cost of the ticket. Once or twice, we even doubled our money.
The lottery is often said to be a tax on the mathematically challenged. That saying is certainly nonsense. Pretty much nobody thinks the odds are in their favor. People who buy lottery tickets do so in hope, desperation, or with a “hey, somebody has to win; why not me?” attitude.
After all, the odds of winning the grand prize in the Powerball is 1 in 292,201,338. Every adult in the European Union could buy a ticket, and they might still miss the winning ticket. I could spend $1,000,000 on each of the twice-weekly Powerball drawings, and it would take me more than five years to get through all the possible combinations.
There are a few things with worse odds. A decade ago here at 3QD we talked about how a well-shuffled deck of playing cards is virtually certain to be in an order never before seen in the history of the universe. I wouldn’t bet on guessing that order. But among everyday things, the odds of winning a big lottery are about as bad as it gets.
Gambling odds favor the house. After all, they wouldn’t offer the game if they didn’t expect to make money over the long run. The odds are ever in their favor. Read more »
Lessons in Chemistry (for Toddlers)
by Ashutosh Jogalekar
I loved chemistry so deeply that I automatically now respond when people want to know how to interest people in science by saying, “Teach them elementary chemistry”. Compared to physics it starts right in the heart of things. —Robert Oppenheimer
How much science can you teach very young children? I have been exploring this question for the past one year or so as I experiment with teaching various kinds of scientific topics to my 3-year-old daughter. She has been generally quite receptive and curious about everything I tell her, and whatever I have failed to teach her is largely a reflection on either the intrinsic difficulties of explaining certain kinds of ideas or my own communication skills.
Chemistry has always been more accessible than physics to laymen, largely because of its colors and explosions and smells and relevance to everyday life; it “starts right in the heart of things”, as Oppenheimer put it. But even at an elemental level it seems easier because of the architectural nature of the subject – atoms which are the building blocks assemble into larger molecules. The basic idea to communicate was to think of atoms as balls that can attach to different numbers of other balls based on what element they represent – one for hydrogen, two for oxygen, three for nitrogen, four for carbon and so on. The combination of atoms into molecules then becomes a simple exercise — just count whether each atom attaches to its right number of neighbors. To make it friendlier, I asked my daughter to see if each atom has the right number of “friends” it holds hands with. Read more »
Bewitched Beasts and Groundhogs
by Jerry Cayford
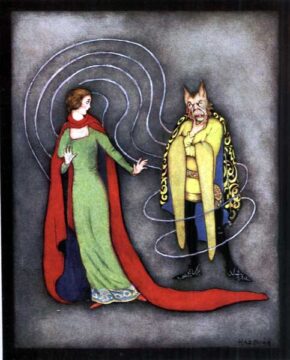
It is almost Groundhog Day again. Time for the ritual rewatching of Groundhog Day, the comically ingenious and wildly successful retelling of “Beauty and the Beast.” More than just a delightful comedy, Groundhog Day does justice to the deep psychological roots of an ancient fairy tale (“Beauty and the Beast” is, along with “Rumpelstiltskin”—about which I wrote previously—in a small group of the oldest stories in Western literature), while reinventing it for our time.
Versions of “Beauty and the Beast” differ so greatly that it is hard to find a core set of elements, or confidently identify what is or isn’t a “version” of the story. (Wikipedia has a long list.) The larger category (ATU 425: The Search for the Lost Husband, or The Animal Bridegroom) includes “Cupid and Psyche,” from ancient Greece and Rome, where the husband is a god, not an animal, and it is Beauty who is tested and must change. Some stories start with marriage instead of ending there. In “East of the Sun and West of the Moon” (Norway), Beauty marries the white bear and only sees her husband as a beast by day. In the dark, though, he comes to her bed as a man, so long as she does not attempt to see his true form.
These tales are tales of alienation between a couple who do not know each other’s secrets, and do not trust each other. They live in material splendor in enchanted castles, and are lonely. The theme is how two become one.
Groundhog Day recreates “Beauty and the Beast” with clever narrative innovations. Usually, Beauty, a paragon of virtue, is the protagonist; but an unchanging protagonist makes for a static story: all the drama, all the change is happening in the secondary character, Beast. By making Beast the protagonist, Groundhog Day brings us close to the thematic action. Another narrative difficulty it solves is how a Beast isolated in a castle has time and reason for profound psychological change. (Fans of Disney’s versions create convoluted analyses about how long the story takes and how time must move differently in the castle and the town.) By giving Beast infinite time, Groundhog Day can thoroughly detail the stages of his transformation from self-centered jerk to caring community member, plausibly earning his release from the spell. Read more »
Perceptions
Sughra Raza. New Wing. November 2023
Digital photograph.
Against Self Improvement: The Negative Capability of Everyday Life
by Chris Horner
Negative Capability, that is, when a man is capable of being in uncertainties, mysteries, doubts, without any irritable reaching after fact and reason… —Keats.
To become mature is to have regained the seriousness one had as a child at play. —Nietzsche
Why do we want to know ourselves? Self knowledge seems like an obvious thing to want, perhaps because ‘the unexamined life is not worth living’, or because self knowledge will make us into better people. Self knowledge, the desire to understand who we are and what we really want can be valuable if it makes us kinder, less prone to arrogant dismissal of others when we see our faults reflected in theirs. Philosophy, psychoanalysis and literature have a lot to do with the pursuit of self knowledge and the self improvement we suppose will accompany it. They seem self evidently good things to want to achieve.
The Trap
Yet sometimes self knowledge can be the wrong thing to aim at. This is when we are dominated by an itch to achieve a stable sense of who we are, or what we ‘really want’ that will bring an end to all that striving. Our myth of personal betterment has a prize glittering before it of the achieved self, the better person we could be, more authentic. The problem here, I’d suggest, is that this itch for the knowledge of the truth about ourselves is a mixed thing: in many ways a valuable part of what we think of as growth and maturity, but also a kind of trap. Read more »
Settle Down!
by Ethan Seavey
I heard:
Why don’t you stop moving around so much? Why do you always bounce your leg/twirl your hair/sit with your legs folded under you/tap your fingers/tap your pen/touch your mustache/hold water in your mouth? Settle down! Why do you play soccer better when you’re rubbing your thumb and forefinger? Why do you sip water out of the side of your mouth so you can still focus on what’s in front of your eyes? Can’t you take a break? Why are you into things (green t-shirts, a long conversation, a movie, a board game) and then suddenly become disinterested? Why are you so frustrated all the time and why can’t you control yourself? Why can’t you focus on what I’m saying? Are you listening, or are you thinking of something else?
And so I thought:
Why am I like this? Why can’t I stop myself from moving? Why am I busted, how am I broken, why doesn’t my brain work?
My energy’s like a wriggling snake and my attention is just one hand. When I grab the head and hold it still, the rattle starts flailing. So I grab the rattle and the head moves again. Your sister hates seeing the head moving (it stresses them out to see the motion of the fangs) and your mother hates the sound of the rattle shaking.
Why am I like this? The snake is a good example but it is inaccurate because it would be more like a bunch of snakes tied in a knot, all wriggling, all needing my attention to stifle their motion. Or a bunch of holes in a field that shoot water and when I cover one hole, the water only flows stronger through the others.
I tried to fix my behavior. At the same time I wanted to stop twirling my hair and bouncing my leg and checking my phone so much. The frustration of not being able to control my body was unbearable (like most frustration to me). I believed that if I tried harder, if I was more disciplined, I could sit still. Read more »
Catspeak
by Brooks Riley