by Ashutosh Jogalekar
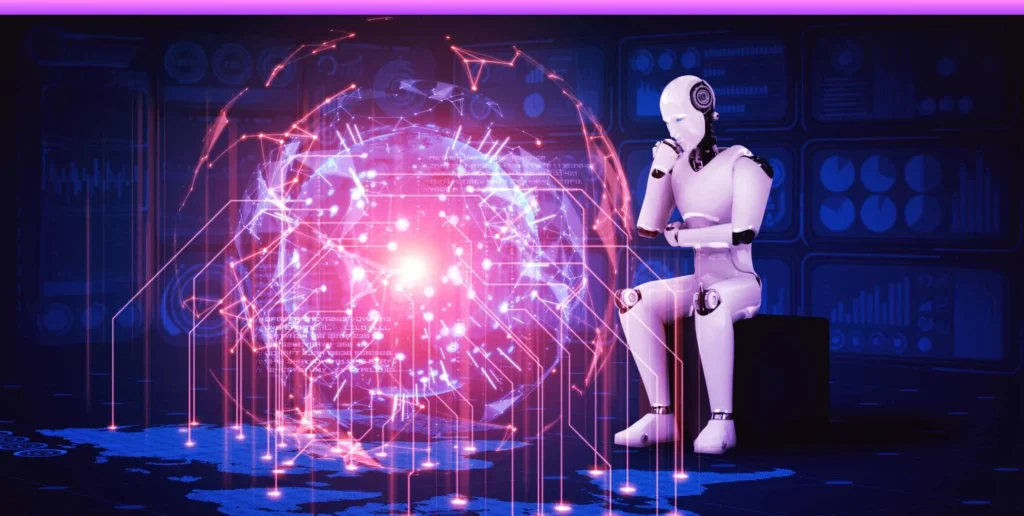
AGI is in the air. Some think it’s right around the corner. Others think it will take a few more decades. Almost all who talk abut it agree that it applies to a superhuman AI which embodies all the unique qualities of human beings, only multiplied a thousand fold. Who are the most interesting people writing and thinking about AGI whose words we should heed? Although leaders of companies like OpenAI and Anthropic suck up airtime, I would put much more currency in the words of Kevin Kelly, a superb philosopher of technology who has been writing about AI and related topics for decades; among his other accomplishments, he is the founding editor of Wired magazine, and his book “Out of Control” was one of the key inspirations for the Matrix movies. A few years ago he wrote a very insightful piece in Wired about four reasons why he believes fears of an AI that will “take over humanity” are overblown. He casts these reasons in the form of misconceptions about AI which he then proceeds to question and dismantle. The whole thing should be dusted off and is eminently worth reading.
The first and second misconceptions: Intelligence is a single dimension and is “general purpose”.
This is a central point that often gets completely lost when people talk about AI. Most applications of machine intelligence that we have so far are very specific, but when AGI proponents hold forth they are talking about some kind of overarching single intelligence that’s good at everything. The media almost always mixes up multiple applications of AI in the same sentence, as in “AI did X, so imagine what it would be like when it could do Y”; lost is the realization that X and Y could refer to very different dimensions of intelligence, or significantly different in any case. As Kelly succinctly puts it, “Intelligence is a combinatorial continuum. Multiple nodes, each node a continuum, create complexes of high diversity in high dimensions.” Even humans are not good at optimizing along every single of these dimensions, so it’s unrealistic to imagine that AI will. In other words, intelligence is horizontal, not vertical. The more realistic vision of AI is thus what it already has been; a form of augmented, not artificial, intelligence that helps humans with specific tasks, not some kind of general omniscient God-like entity that’s good at everything. Some tasks that humans do will indeed be replaced by machines, but in the general scheme of things humans and machines will have to work together to solve the tough problems. Which brings us to Kelly’s third misconception.
The third misconception: A super intelligence can solve our major problems.
As a scientist working in drug development and biotechnology, this fallacy is my favorite. Just the other day I was discussing with a colleague how the same kind of raw intelligence that produces youthful prodigies in physics and math fails to do so in highly applied fields like drug discovery: when was the last time you heard of a 25-year-old inventing a new drug mainly by thinking about it, the way a 25-year-old Werner Heisenberg invented quantum mechanics while recovering from hay fever on an island? An analysis of Nobel Prize winners shows that the mean age at which a Nobel Prize is awarded increases from physics through chemistry to medicine. That is why institutional knowledge and experience counts in fields like medicine and drug discovery, and that’s why laying off old timers is especially a bad idea in these fields.
In case of drug development, the reason why a young hotshot scientist is unlikely to conjure up a new drug seems clear: it’s pretty much impossible to figure out what a drug does to a complex, emergent biological system through pure thought. You have to do the hard experimental work, you have to find the right assays and animal models, you have to know what the right phenotype is, you have to do target validation using multiple techniques, and even after all this, when you put your drug into human beings you go to your favorite church and pray to your favorite God. The whole field is a graveyard of failed ideas and perhaps has the highest attrition rate of any applied science field. None of its major challenges can be solved by just thinking about it, no matter what your IQ. Kelly calls the belief that AI can solve major problems just by thinking about it “thinkism”: “the fallacy that future levels of progress are only hindered by a lack of thinking power, or raw intelligence.”
Rather:
“Thinking (intelligence) is only part of science; maybe even a small part. As one example, we don’t have enough proper data to come close to solving the death problem. In the case of working with living organisms, most of these experiments take calendar time. The slow metabolism of a cell cannot be sped up. They take years, or months, or at least days, to get results. If we want to know what happens to subatomic particles, we can’t just think about them. We have to build very large, very complex, very tricky physical structures to find out. Even if the smartest physicists were 1,000 times smarter than they are now, without a Collider, they will know nothing new.”
To which I may also add that no amount of Big Data will necessarily translate to the correct data.
Kelly also has some useful words to keep in mind when it comes to computer simulations, and this is another caveat for drug discovery scientists:
“There is no doubt that a super AI can accelerate the process of science. We can make computer simulations of atoms or cells and we can keep speeding them up by many factors, but two issues limit the usefulness of simulations in obtaining instant progress. First, simulations and models can only be faster than their subjects because they leave something out. That is the nature of a model or simulation. Also worth noting: The testing, vetting and proving of those models also has to take place in calendar time to match the rate of their subjects. The testing of ground truth can’t be sped up.”
Most molecular simulations for instance are fast not just because of better computing power but because they are intrinsically leaving out parts of reality – and sometimes significant parts (that’s the very definition of a ‘model’ in fact). And it’s absolutely true that even sound models have to be tested through often tedious experiments. Molecular Dynamics (MD) simulations in which proteins and other molecules are made to wiggle and jiggle are good examples. You can run an MD simulation very long and hope to see all kinds of interesting fluctuations emerging on your computer screen, but the only way to know whether these fluctuations (a protein loop moving here, a pocket on a protein that could potentially bind to a drug transiently opening up there) correspond to something real is by doing experiments which are expensive and time-consuming. Many of those fluctuations from the simulation may be irrelevant and may lead you down rabbit holes. In addition, in the real world proteins are part of networks of other proteins and are buffeted by all kinds of proteins and other molecules – ions, other proteins, hormones and of course, the water that is ubiquitous in our body. There’s no getting around this bottleneck in the near future even if MD simulations were to be sped up another thousand fold. The problem is not one of speed, it’s one of not being able to capture all of reality.
The fourth misconception: Intelligence can be infinite.
Firstly, what does “infinite” intelligence even mean? Infinite computing power? The capacity to crunch an infinite amount of data? Growing infinitely along an infinite number of dimensions? Being able to solve every single one of our problems ranging from nuclear war to marriage compatibility? None of these tasks seems even remotely within reach in the near or far future. There is little doubt that AI will keep on crunching more data and keep on benefiting from more computing power, but its ultimate power will be circumscribed by the laws of emergent physical and biological systems that are constrained by the hard work of experiment and various levels of understanding.
AI will continue to make significant advances. It will continue to “take over” specific sectors of industry and human effort, mostly with little fanfare. The mass of workers it will continue to quietly displace will pose important social and political problems and we are right to worry about and try to prepare for it. But from a general standpoint, AI is unlikely to take over humanity, let alone destroy it. Instead it will do what pretty much every technological innovation in history has done: keep on making solid, incremental advances that will both improve our lives and create new problems for us to solve.