by Jochen Szangolies
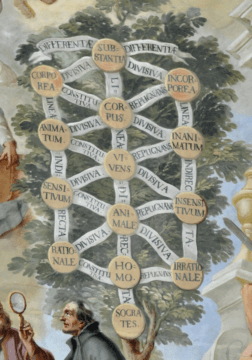
The arbor porphyriana is a scholastic system of classification in which each individual or species is categorized by means of a sequence of differentiations, going from the most general to the specific. Based on the categories of Aristotle, it was introduced by the 3rd century CE logician Porphyry, and a huge influence on the development of medieval scholastic logic. Using its system of differentiae, humans may be classified as ‘substance, corporeal, living, sentient, rational’. Here, the lattermost term is the most specific—the most characteristic of the species. Therefore, rationality—intelligence—is the mark of the human.
However, when we encounter ‘intelligence’ in the news, these days, chances are that it is used not as a quintessentially human quality, but in the context of computation—reporting on the latest spectacle of artificial intelligence, with GPT-3 writing scholarly articles about itself or DALL·E 2 producing close-to-realistic images from verbal descriptions. While this sort of headline has become familiar, lately, a new word has risen in prominence at the top of articles in the relevant publications: the otherwise innocuous modifier ‘general’. Gato, a model developed by DeepMind, we’re told is a ‘generalist’ agent, capable of performing more than 600 distinct tasks. Indeed, according to Nando de Freitas, team lead at DeepMind, ‘the game is over’, with merely the question of scale separating current models from truly general intelligence.
There are several interrelated issues emerging from this trend. A minor one is the devaluation of intelligence as the mark of the human: just as Diogenes’ plucked chicken deflates Plato’s ‘featherless biped’, tomorrow’s AI models might force us to rethink our self-image as ‘rational animals’. But then, arguably, Twitter already accomplishes that.
Slightly more worrying is a cognitive bias in which we take the lower branches of Porphyry’s tree to entail the higher ones.
This is often a valuable shortcut (call it the ‘tree-climbing heuristic’): all animals with a kidney belong to the chordates, thus, if you encounter an animal with a kidney, you might want to conclude that it is a chordate. The differentia ‘belongs to the phylum chordata’ thus occupies a higher branch on the Porphyrian tree than ‘has a kidney’. But there is no necessity about this: a starfish-like animal (belonging to the phylum echinodermata) with a kidney seems possible in principle, even if none is known. Lumping it in with the chordates on the basis of its having a kidney would thus be a mistake—the heuristic misfires.
Something of this sort is, I think, at the root of recent claims that LaMDA, a chatbot AI developed by Google, has attained sentience. The reasoning appears to be the following: LaMDA exhibits (some trappings of) intelligence, intelligent beings are sentient (the tree-climbing heuristic: all intelligent beings we know—i. e. humans—are sentient), hence, LaMDA is sentient. Whether this logic is sound then depends on whether it is possible to be intelligent, yet not sentient.
It is here that, I think, the real—and too often passed-over in silence—issue hides. We typically think of intelligence as a comparatively uncontroversial notion: as opposed to sentience, or consciousness, the consensus seems to be that intelligence can be readily assessed ‘from the outside’. It is a behavioral notion—intelligent is as intelligent does, to subvert the classic quote from Forrest Gump. Examining the behavior of a system should thus allow us to judge it intelligent. But things may be more subtle.
Intelligence Everywhere (And Nowhere)
The history of AI is replete with squabbles over what computers can or can’t do, and subsequent accusations of shifting the goalposts. When we posit that a computer, lacking intelligence, cannot perform some task X (say, play a competent game of chess), and later on, a computer successfully does perform it, there seem to be two general patterns of response: an ‘accumulative’ view that considers X to be a task added to the portfolio of computer capabilities, and a ‘reductive’ view that considers X being performed by computer a deflation of the claim that you need intelligence for X. Thus, a computer learning to play chess is either one step closer to the goal of possessing ‘real intelligence’, or exposes the problem of competent chess-playing to be solvable by ‘mere automation’, without any of said ‘real intelligence’.
Both positions are easily parodied. On one end of the spectrum, the hapless technophile drinks up the latest hype from billion-dollar companies maximizing their market value; on the other, the hopeless romantic yearns for a unique place for humanity, some remnant of our ‘special’ nature already so diminished by the discoveries of Kepler or Darwin.
However, both seem to me mistaken in a similar way: at the heart of each, there is an assumption that there is some fixed point where ‘general intelligence’ resides. Either, the progress of AI shows then a path towards that point, to be walked down step by step; or, it shows that whatever general intelligence is, it’s not that, with ‘that’ being the latest AI accomplishment replicated across breathless headlines in the relevant outlets.
But then, what actually is general intelligence? The problem has existed longer than the idea of intelligent machinery: feats routinely associated with intelligence are replicated throughout the animal, plant, and even fungi kingdom. Octopuses, dolphins, and some non-human primates are familiar examples of animal smarts; more surprisingly, crows show signs of stunningly high intelligence.
However, these are no threat to our intuitive notion of intelligence: so, some animals are cleverer than we thought. Still, this is a difference in quantity—not in quality. It’s the same sort of intelligence we have, just maybe a bit less.
This stance becomes harder to maintain once we consider more exotic manifestations of (apparent?) intelligence. Consider the complex ‘societies’ formed by ants and similar state-forming insects. Their sophistication seems to belie complex reasoning to the point that, in 1879, Sir John Lubbock inserted a microphone into an anthill with the intent to ‘ascertain whether the ants themselves produced any sounds for the purpose of conveying signs or ideas’—figure out if ants talk to each other.
To the extent that ants ‘talk’, they do so not with sounds, but rather, with pheromones—but the notion is still somewhat misleading: to talk suggests that there are ideas communicated that originate with the talkers; but the individual ant is not the locus of ant intelligence. Rather, with ants, we are met with an intelligence that manifests itself on an intersubjective level: what intelligent acts are performed by ants are due to the hive—the superorganism—rather than due to individual ants. Here, then, we meet a more substantive challenge to our usual notion of intelligence, one, unlike human rationality, not centered on the individual, but distributed across a collective. Thus, groups may possess an intelligence not limited by that of their members—and, if groups aren’t sentient (which we have no reason to assume), here we have a counterexample to intelligence implying sentience.
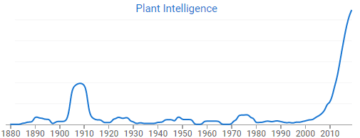
But we can go further, leaving the animal kingdom behind. While plants are traditionally regarded as passive, sessile beings, in recent years, ‘a revolution is sweeping away the detritus of passivity, replacing it with an exciting dynamic—the investigation of plant intelligence is becoming a serious scientific endeavour’, as the matter is put by molecular biologist Anthony Trewavas. As an example, a 2016 experiment carried out by a research team led by Monica Gagliano from the University of Western Australia has even produced evidence that the humble garden pea is capable of learning the association between a light-based cue and the location of a food source.
The idea is not an entirely new one, however—already in 1880, Charles Darwin, together with his son Francis, published The Power of Movement in Plants, notable for proposing the ‘root-brain hypothesis’ that sees the apices of plant roots as analogous to the animal brain.
Possible examples of intelligent behavior exist even farther from the anthropocentric paradigm of big-brained animals carrying out solitary intellectual exercises. In a much-publicized experiment, the humble slime mold was shown to be able to solve certain optimization problems, approximating the structure of Tokyo’s railway system. Bacteria use quorum sensing to adapt their behavior to changes in population density by modifying their own gene expression. Indeed, intelligence even seems to act across borders of species and huge scales of time—witness the temptation to see evidence of ‘intelligent design’ in the results of evolutionary selection.
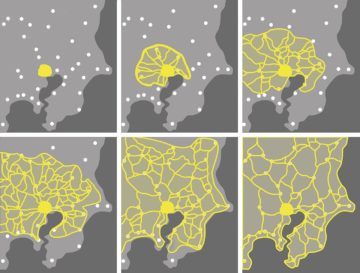
What is the right conclusion to draw, here—that intelligence is far more widespread than one might have assumed, or that thus, none of these behaviors need ‘real intelligence’ after all? Both options present a gradualism problem: accepting the first, it becomes challenging to find behaviors that are not intelligent, while accepting the latter, we seem hard pressed to identify those that are. (Could not the building of cities, roads, and the like be simply due to some sophisticated, but mechanical instinct, only some million more iterations of the same evolutionary logic that leads ants to construct their complex and climate-controlled hives?)
What Does The Turing Test Test For, Anyway?
Perhaps, you might argue, the apparent trouble is just a bit of sleight of hand on my part: while talking a great deal about supposed examples of intelligence, I have so far neglected to tell you what I think intelligence is. In part, you’re right to suspect a strategy: we all come with different preconceived notions of intelligence, and thus, I hope, different reactions to the above examples: while ant intelligence might strike you as absurd, perhaps you don’t have similar reservations about plant intelligence; while slime molds clearly just react mechanically, crows are properly rational, if maybe less so than humans. (This brings to mind the alleged quote of a Yosemite Park forest ranger on the problem of designing a bear-proof garbage can: “There is considerable overlap between the intelligence of the smartest bears and the dumbest tourists.”)
What I hope the above has accomplished is to foster a sense that the notion of intelligence is more fuzzy than is usually recognized. By extension, then, so is the notion of general intelligence. But here, we are often told, there is an unambiguous means for recognizing its presence: the much-vaunted Turing Test, in which an ‘imitation game’ is proposed, whose object it is to decide which of the two partners in a conversation is a human, and which a machine.
To his great credit, Turing, much more so than some of his later followers, sees the difficulties of defining ‘intelligence’ (or ‘thinking’), and proposes to do an end-run around it, thus operationalizing a challenging problem in the form of a simple test. However, this solution is not without its problems.
First, by picking an explicitly anthropocentric frame of reference, one might worry that certain forms of intelligence—be it that of anthills or aliens—are excluded from the start. But the more immediate question is whether the test as conceived actually accomplishes its own stated goal: is the statistical inability of distinguishing between a human and a machine interlocutor actually indicative of, well, anything? And if so, of what?
A point against the sufficiency of the Turing Test is the ‘humongous table’-argument. Imagine the internal dynamics of the machine to be given by nothing but an enormous list of input-output pairs, with the input being all possible conversations (up to the length needed to ‘pass’ the test, which might be arbitrarily big, but is guaranteed to be finite), and the output a sensible reply. Such a system would pass the Test by construction; but the only ‘intelligence’ it possesses is that of matching the conversation up to a certain point with the set of input-strings.
One might hold that this is cheating: after all, the table must have been constructed, somehow; if it has been constructed by an intelligent being (human, machine, or other), then it’s really that being that’s being tested, by the somewhat unusual proxy of telling all their conceivable answers to a machine beforehand. But the table need not have come about in this way: suppose we start with a program capable of passing the Test; then, we can apply mechanical transformation rules to this program to gradually transform it into an appropriate table. If the table-based program is not intelligent, then at what point is the intelligence lost?
Contrariwise, we might start with the table, and reduce its size, taking advantage of the huge amounts of redundancy bound to be present. At some point, we are left with a program that has no obvious ‘lookup table’ structure anymore, but rather, a great deal of sophisticated processing to generate an answer—have we somehow ‘created’ intelligence in the process of compressing the table?
Again, it seems, we are faced with a problem of gradualism: where does intelligence end, and where does it begin? Certainly: the vagueness of a concept does not constitute a refutation of its usefulness. But it does change the spectrum of possible answers we can expect: rather than clear-cut distinctions between intelligent and non-intelligent systems, we are left with varied, competing, and perhaps even inconsistent judgments. Does it then make sense to expect the sort of unambiguous endpoint the concept of a ‘general intelligence’ seems to imply?
AIXI And More General Intelligence
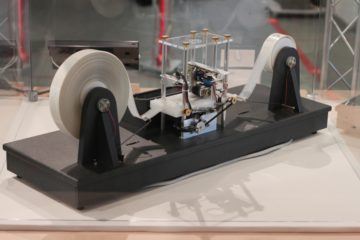
Perhaps somewhat surprisingly, given the above discussion, there actually exist mathematically precise formulations that can lay claim to a ‘general’ sort of intelligence. One example is the AIXI agent, proposed by AI researcher Marcus Hutter. Given an arbitrary problem, it can be shown to perform asymptotically as well on that problem as a special-purpose problem solver would: it is a general problem-solver in this sense.
While one might quibble with its ‘problem-centred’ approach to defining intelligence, the point I want to make here is a different one. Conceding that this is a sensible delineation of rationality, we find that still, it lies beyond our grasp: the theory on which AIXI is built, Solomonoff induction (a systematic way of generating and updating hypotheses about the world), is uncomputable—meaning that it cannot be implemented on any computer, no matter how powerful. Moreover, any theory equivalent in power to it must be uncomputable, too.
This means that if AIXI is a formalization of general intelligence, we can never actually implement it—or, in other words, human intelligence either lies beyond the power of any computer, or it can’t be general. While I believe that, in general, the human mind is not a computer program, I see no reason to believe that human intelligence is capable of any feats that cannot be equaled, at least in principle, by a computer. In fact, I don’t believe there are physical processes that can be exploited to ‘compute’ more than possible using a regular computer (given enough time and memory).
The point where general intelligence resides then is a vanishing point; all concretely instantiated intelligences can only occupy different points on the manifold of lines converging towards it. No intelligence is general, but any given intelligence may be more general than another. The reason for the vagueness of the concept of intelligence is that its absolute end-point lies forever beyond reach.
There might be cause for some concern, here. Most worries about the technological singularity, the AI apocalypse, and so on, focus on intelligences exceeding ours in terms of quantity: doing the same things we do, merely faster and better. But if human intelligence is not general, there may be intelligent behaviors that we are unable to even recognize. An intelligence descending the Porphyrian Tree via a different route than ours—substance, corporeal, mechanical, computational, intelligent or even substance, corporeal, living, alien, intelligent—might differ from ours not merely in power, but in kind, to a degree that we might fail to recognize it. To us, its behavior might then seem irrational, arbitrary, even random.
This complicates the task of AI safety: there are strategies a runner might use to not be caught by a faster runner—creating obstacles, taking shortcuts, and so on. But what if their opponent suddenly develops the ability to fly?