Jerry Seinfeld is fond of comparing jokes to machines: Jokes are tiny intricately crafted machines, where all the parts fit neatly and precisely together, moving in precise, if sometimes surprising, fashion. Last summer I decided to pit Seinfeld against the precisest (is that even a word?), most super-modern, and biggest intricate machine I could think of, GPT-3. You may have heard of it, it’s an AI engine. As you may know, AI engines are not at all like automobile engines. They’re not mechanical devices. They’re, well, you know, they live up in the cloud, where all the super-modern high-tech gizmos and gadgets hang out, or whatever it is that they do while secretly plotting to take over the world.
Seinfeld tells a joke
But we’re getting ahead of ourselves. Let’s start with something we know, Jerry Seinfeld. Here’s his very first television appearance. It is from 1977 on Celebrity Cabaret, a nationally syndicated show. He’s doing a bit that involves the Roosevelt Island tramway. You know what that is? If you’re familiar with the New York City area you do. Otherwise, it may be something of a mystery.
But first things first. Here’s the video (joke starts at about 0:29):
I’m not sure he’d get away with the word “ghetto” these days, but back in the 1970s it was A-OK. This particular sketchy part of town is the South Bronx which, as my colleague Michael Liss reminded me, was the setting for a 1981 crime drama, Fort Apache, The Bronx. It’s also the setting for the 2016 Netflix series, The Get Down, about the early days of hip-hop.
Here’s the bit as Seinfeld listed it in his recent book, Is This Anything? (2020), where he lists all his bits in the order he performed them in his career:
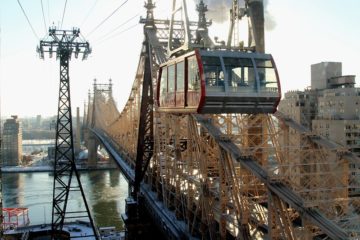
Photo by Thank You (22 Millions+) views
I see they just finished the Roosevelt Island Tramway.
That’s nice…
The city’s going bankrupt,
they’re putting up rides for us.
Next thing you know, there’ll be a roller coaster through the South Bronx.
That would be the first roller coaster where the people scream on the flat part of the ride.
You may or may not think that’s funny. If you do, you don’t need to be told way. If you don’t, you probably know why it’s supposed to be funny even if you don’t think it is.
Just to be sure we’re on the same page, though, I’m going to explain it anyway.
When do you scream on a roller coaster? You scream at the top, after the car has come over the hump and starts going down. That’s the scary thrilling part of the ride. Once you hit bottom the ride isn’t so thrilling. But if you’re in a sketchy part of town where thieves and muggers and hookers and dope dealers and three-card Monte players and who knows what other kind of riff-raff are hanging out, if the flat part of the ride is in that country, then it’s pretty scary and so you scream.
That’s all obvious. To you. But does GPT-3 know it? Let’s find out.
Just what is GPT-3?
GPT-3 is a massive AI engine that OpenAI revealed in June 2020. Massive? It’s got 175 billion parameters. Parameters? Don’t worry about it. Think of a parameter as a control knob on your TV – do TV’s have knobs anymore? Imagine a TV with 175 billion, with a “B”, knobs. That’s a lot of twiddling. It was trained on 499 billion tokens (think, roughly, words) of text sucked in from the internet. So it has processed text about Roosevelt Island, tramways, roller coasters, the South Bronx and a lot of other things, such as Antarctica, green cheese, Mata Hari, redwoods, giant Komodo dragons, fried rice, the Saturn V rocket, Jerry Seinfeld, pencils, The Tale of Genji, whales, catapults, looms, fruit flies, and anything else laying around on the Internet.
What GPT-3 does is to produce text. You feed it a bit of text and it takes it and runs with it by producing a continuation of the text. The text it produces is often astonishingly natural. It seems like a human wrote it. But no human did, just a dumb, but huge, ginormous, machine.
If you feed GPT-3 some text and then ask a question, it will answer the question. That’s what I did with GPT-3. Well, not me, my friend Phil Mohun is the one who quizzed the machine. He gave it Seinfeld’s bit, and then asked a question. GPT-3 answered. Since I was particularly interested in the last line, the punch line, that’s what Phil asked about.
GPT-3 explains Seinfeld’s joke
1] Here’s the first interaction. I’ve set Phil’s words in boldface.
Jerry Seinfeld tells the following joke:
I see they just finished the Roosevelt Island Tramway.
That’s nice…
The city’s going bankrupt,
they’re putting up rides for us.
Next thing you know, there’ll be a roller coaster through the South Bronx.
That would be the first roller coaster where the people scream on the flat part of the ride.
Q: Why would the people scream on the flat part of the ride?
GPT-3: Because it’s the Bronx.
Well, yeah. But just what is the significance of that fact? I wanted to see if GPT-3 understood the relationship between being scared on a roller coaster and being scared on the ground in the South Bronx. GPT-3’s answer doesn’t tell me what, if anything, it “knows” about either roller coasters or the South Bronx.
Note: GPT-3 doesn’t actually know anything at all. But let’s skip that for now and just watch how it behaves. We’ll return to this at the end.
So I asked Phil to give it another go.
2] He increases the “temperature.” Don’t worry about just what that is, but increasing the temperature raises the amount of randomness in GPT-3’s response. Note that on this try Phil also gave GPT-3 the whole bit, as in example 1. That’s true of the other examples as well, but I’m omitting that part because it’s redundant. Just assume GPT-3 got the whole deal.
Q: Why would the people scream on the flat part of the ride?
GPT-3: There’ll be nothing to see for miles but rubble.
We’re getting somewhere. It seems like GPT-3 doers “knows” something about the South Bronx. But what does rubble have to do with screaming?
3] Here’s another response:
Q: Why would the people scream on the flat part of the ride?
GPT-3: So the people in Queens can see them.
That’s very interesting, and indicates some knowledge, excuse me, “knowledge,” of geography as Queens and the South Bronx adjoin one another. But the roller coaster would have to be pretty close to Queens in order for the screams to reach people there. How far does GPT-3 think screams can be heard, under what wind conditions, and so forth. It’s not clear what’s going on inside GPT-3 – why would people on the roller coaster want to be noticed by people in Queens? – but the reply is not flat-out nonsense. It’s just a bit off.
4] Let’s give GPT-3 another chance.
Q: Why would the people scream on the flat part of the ride?
GPT-3: The ride is in the South Bronx.
Q: What about the South Bronx would cause people to scream?
GPT-3: Look at a map. It’s all twists and turns—like a roller coaster!
Now we’ve got a two-turn interaction with GPT-3. Phil follows GPT-3’s first answer with another question.
Did GPT-3 actually look at a map? I think not. The only thing it “looked at” during training was those tokens, mere husks of words. Still, I don’t see why there wouldn’t have been some text somewhere in the database that gave it some idea about the geography. Not, mind you, that I’m at all clear about the geography myself; I’ve only been to the South Bronx once and that was in and out, near the Grand Concourse. Still, I score this as a good response.
5] Let’s go for another response to the same prompt:
Q: Why would the people scream on the flat part of the ride?
GPT-3: The ride is in the South Bronx.
Q: What about the South Bronx would cause people to scream?
GPT-3: You’d have to be there.
A variant of the joke that interacts with the South Bronx’s reputation for high crime rates and arson.
In 1982, the South Bronx was experiencing a crisis of arson, fires, and abandonment. By the end of that year, the city would have paid out $10 million to local contractors for repairs and maintenance.
Whoa! I love this. GPT-3 really dug down and found out something about the South Bronx, something directly relevant to the bit.
You might be wondering, however, why GPT-3 gave such a different response to #5 than #4 when the prompts are the same. GPT-3 is not deterministic. That is to say, when given the same input more than once it doesn’t necessarily give the same output. Not that it’s got a mind – at least I’m not going to credit it with one, and I don’t see why anyone would. But it’s complicated. Frankly, we don’t really know what it’s doing.
Let’s take a couple more spins on this merry-go-round.
6] Notice that Phil’s second question is explicitly about screaming:
Q: Why would the people scream on the flat part of the ride?
GPT-3: The ride is the South Bronx.
Q: Why do people scream on roller coaster rides?
GPT-3: Because that’s the only time of the ride they realize there are other options.
That’s hilarious! What other options? Options to what? What does screaming have to do with options? This makes no sense at all. I wonder if Seinfeld could somehow work it into the bit.
7]
Q: Why would the people scream on the flat part of the ride?
GPT-3: The ride is the South Bronx.
Q: Why do people scream on roller coaster rides?
GPT-3: Because they’re having fun!
8]
Q: Why would the people scream on the flat part of the ride?
GPT-3: The ride is the South Bronx.
Q: Why do people scream on roller coaster rides?
GPT-3: Because it’s scary.
These last two are very interesting. Both make sense and are, I believe, true. And yet they are somewhat at odds with one another, no? Roller coaster rides are both fun and scary. In fact they are fun because they are scary, scary but not dangerous. Does fun always have to be based on something scary? I think not. Are scary things always fun? Certainly not. I wonder how much of that GPT-3 understands? For that matter, how much about that do we humans understand? Not as much as we’d like.
And with that I decided we’d questioned GPT-3 enough. I’m pretty sure such a Q and A could have gone on for a while. I don’t know where it would have gone but I’m pretty sure GPT-3 would not have “figured out” the tricky relationship between scariness and fun.
Why not? Because GPT-3 can’t “figure out” anything at all. But it can put up an interesting front.
A different frame
I could have framed those interactions differently. I could have told you that I showed the video to some person I met on the street and then asked them about it. Thus:
Alice:
Bill B: Why would the people scream on the flat part of the ride?
Alice: So the people in Queens can see them.
Franklin:
Bill B: Why would the people scream on the flat part of the ride?
Franklin: The ride is the South Bronx.
Bill B: Why do people scream on roller coaster rides?
Franklin: Because that’s the only time of the ride they realize there are other options.
What would you have thought then? Would you have guessed that these aren’t people at all, but a computer? Or would you simply have thought that these were some odd people? If I’d disguised all of these interactions as conversations between me and various individuals you likely would have wondered how I’d managed to find so many odd and strange people. But if you just saw the Alice interaction or the Franklin interaction, what would you have thought?
Order and chaos in strings of words
We experience language as physical symbols, sound in the case of speech, script or print in the case of writing. But, as you know from Linguistics 101, word meanings aren’t there in the physical symbol. They’re in our heads. We automatically match meaning with physical symbol when we listen or read. That is, we are interpreting the physical symbol. If the interpreted meaning makes sense, we think nothing of it. If it doesn’t, then we work at it, reasoning and guessing, until we have a plausible account.
That’s what we were doing in trying to figure out what GPT-3 was up to. Since we knew, however, that GPT-3 is not human, we didn’t work too hard. And yet there’s enough more or less intelligible order in GPT-3’s responses to be, you know, interesting, puzzling.
As I already pointed out, GPT-3 doesn’t actually know anything. It was trained by analyzing swathes and acres and oceans of text sucked in off the web. Those texts are just naked word forms and, as we’ve observed, there are no meanings in word forms. GPT-3 is able to compute relationships between word forms as they appear, one after the other, in linguistic strings, and from that produce a simulacrum of sensible language when given a cue.
How could that possibly work? Consider this passage from a famous memorandum (PDF) written by the scientist and mathematician Warren Weaver in 1949 when he was head of the Natural Sciences division of Rockefeller Foundation:
If one examines the words in a book, one at a time as through an opaque mask with a hole in it one word wide, then it is obviously impossible to determine, one at a time, the meaning of the words. “Fast” may mean “rapid”; or it may mean “motionless”; and there is no way of telling which.
But if one lengthens the slit in the opaque mask, until one can see not only the central word in question, but also say N words on either side, then if N is large enough one can unambiguously decide the meaning of the central word. The formal truth of this statement becomes clear when one mentions that the middle word of a whole article or a whole book is unambiguous if one has read the whole article or book, providing of course that the article or book is sufficiently well written to communicate at all.
That’s a start, and only that. But, by extending and elaborating on that, researchers have developed engines that weave elaborate webs of contextual information about relations between word forms. It is through such a web – in billions of dimensions! – that GPT-3 has been able to create an uncanny simulacrum of language. Hanging there, poised between chaos and order, the artificial texts tempt us into meaning.
* * * * *
Here’s a 3QD post about Seinfeld’s craft. Here’s one about Seinfeld and Obama. And here’s a working paper from 2016, Jerry Seinfeld & the Craft of Comedy.
A link to my Seinfeld posts at New Savanna.
For extended remarks on GPT-3, see my working paper, GPT-3: Waterloo or Rubicon? Here be Dragons, Version 2, Working Paper, August 20, 2020, 34 pp., https://www.academia.edu/43787279/GPT_3_Waterloo_or_Rubicon_Here_be_Dragons_Version_2.