Adam Garfinkle in Inference Review:
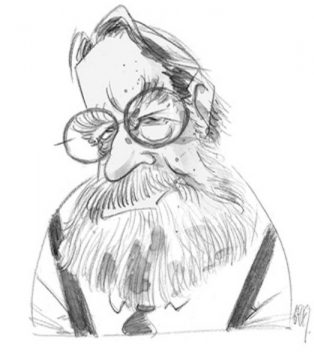
THERE HAVE BEEN fakes as long as there have been frauds, and that is a very long time; but deepfakes are new fakes, and having initially loitered along the margins of general awareness, they are now occupied in haunting it. Tens of thousands of deepfakes have already been created. The technical means of fiddling with images is hardly new. Standing beside Joseph Stalin in one photograph taken along the newly completed White Sea Canal, Nikolai Yezhov disappeared from the very same photograph some months later, as he, in fact, had disappeared from life. The fakery is fine, but it is no better than that, the ensuing photograph visually unbalanced by a lot of gray canal water where Yezhov had once stood. It is thanks to a technology invented in 2014 that deepfakery is capable of taking verisimilitude to a new level.
THE ABILITY TO produce ever more persuasive deepfakes has been made possible by a recent form of machine learning called generative adversarial networks—or GANs. A GAN operator pits a generator (G) against a discriminator (D) in a gamelike environment in which G tries to fool D into incorrectly discriminating between fake and real data. The technology works by means of a series of incremental but rapid adjustments that allows D to discriminate data while G tries to fool it.
How fast are these adjustments? Very fast. A computer can play 24 trillion games of Texas Hold’em every second. To beat human opponents, a computer does not need to assess their strategies. It relies on the patterns it picks out, and assumes only that human strategy is limited to a few flexible tactics. DeepMind beat human players at 99.8% of StarCraft II games, a game subtler and more abstract than Texas Hold’em.
More here.