by Robyn Repko Waller
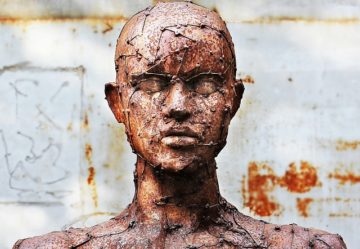
AI has a proclivity for exaggeration. This hallucination is integral to its success and its danger.
Much digital ink has been spilled and computational resources consumed as of late in the too rapidly advancing capacities of AI.
Large language models like GPT-4 heralded as a welcome shortcut for email, writing, and coding. Worried discussion for the implications for pedagogical assessment — how to codify and detect AI plagiarism. Open-AI image generation to rival celebrated artists and photographers. And what of the convincing deep fakes?
The convenience of using AI to innovate and make efficient our social world and health, from Tiktok to medical diagnosis and treatment. Continued calls, though, for algorithmic fairness in the use of algorithmic decision-making in finance, government, health, security, and hiring.
Newfound friends, therapists, lovers, and enemies of an artificial nature. Both triumphant and terrified exclamations and warnings of sentient, genuinely intelligent AI. Serious widespread calls for a pause in development of these AI systems. And, in reply, reports that such exclamations and calls are overblown: Doesn’t intelligence require experience? Embodiment?
These are fascinating and important matters. Still, I don’t intend to add to the much-warranted shouting. Instead, I want to draw attention to a curious, yet serious, corollary of the use of such AI systems, the emergence of artificial or machine hallucinations. By such hallucinations, folks mean the phenomenon by which AI systems, especially those driven by machine learning, generate factual inaccuracies or create new misleading or irrelevant content. I will focus on one kind of hallucination, the inherent propensity of AI to exaggerate and skew.
Here I don’t mean exaggeration, skew, or bias of a human garden-variety. I’m not referring to the encoded racism of COMPAS from the data set or the misogynistic images of AI art, a phenomenon often explained by appeal to the explicit or proxy (human) social bias in the training set. This kind of bias with AI is detectable, insofar as it is interpretable, or in terms or a form understandable by humans.
Instead, the kind of exaggeration we ought to worry more about is more akin to the much-hypothesized-about unrealistic hands in AI art. Until a few weeks ago, one rule-of-thumb (pun intended) give-away for AI-generated visual content was hands. Six-plus digit, extra tangled human hands. This is reminiscent of the extra-leggy AI spiders a few years prior and still. Notice, though, that we haven’t taught AI that spiders have more than eight legs. They don’t. Or that human hands are (typically) tangled. This kind of representation of spiders and humans isn’t coming from the photographs we feed in. Likewise, some users of the popular portrait-creating LENSA a few months back reported misogynistic and distorted body images, curiously generated from head-only selfies as training images.
One could take this erroneous AI output as an instance of a so-termed hallucination in AI. But what is its source? Not the images that we feed in. Local features such as pixel color and location, and labels of HUMAN and SPIDER on photos of human and spider bodies don’t exhibit these exaggerations or any related tracker features for it. Rather, the AI system must be introducing these exaggerations. (In fact, I asked ChatGPT to explain why AI hallucinates extra-leggy spiders. Its response is illuminating.)
Visual AI output, then, highlights that in the case of some AI hallucinations, AI is introducing exaggerations and bias even where none exists in the training set. Exaggeration and bias alien to our own. But how or why is such exaggeration introduced?
As I’ve mentioned prior, what marks out any machine learning from mere statistics is that machine learning programs use initial features and patterns (of patterns of patterns) of features in training data to construct new features that offer better predictions or utility for the task. Deep learning is but one type of machine learning. A multitude of cross-cutting approaches and techniques fall under the machine learning umbrella, including, but not limited to, methods of classification, regression, clustering, visualization, and projection, and methods such as decision trees, random forests, gradient boosting, and support vector machines. What is distinctive, then, about machine learning is its procedure of taking features defined at some stage as a basis for constructing more defining features for the task (image recognition, object detection, social classification), and so on, iteratively. Let’s call these assembled features. Features assembled by the program. Assembled features are what make machine learning so powerful. At Big Data pattern detection. At innovation in output. At enhanced predictive accuracy.
Assembled features, however, are the key to explaining the artificial hallucination of exaggeration in AI output. Any created exaggeration or bias must emerge in this high-dimension, highly reconfigured, dynamic representational space of the AI system. Indeed, the process of constructing assembled features is inherently prone to hallucinatory exacerbation of certain patterns. (See here for an in-depth discussion of why assembled features produce exaggeration and bias.)
Now in the case of the extra-leggy spiders or gnarled human hands, we can recognize these hallucinations in a way that AI cannot. We can see that the AI has constructed an exaggerated representation of spiders and hands. Then we might correct for them, as was the case for, for instance, Midjourney and the human hands. Likewise for many factual errors in ChatGPT: One can safeguard against artificially hallucinated citations and claims in your GPT output with some good-old-fashioned fact-checking. She does not work at University of Florida. No book exists by that title. Etc.
But note that this exaggeration is inherent to any machine learning. Machine learning systems will construct features and so exaggerate among patterns of data for any data set. (For a demonstration, see here.) And therefore machine learning application to any domain will contain exaggeration of the represented as a hallucination, be it social data (suitable job candidates), financial data, text or images.
Now we cannot always tell the difference between AI and human output — take the relative failure of algorithms designed to weed out AI-written essays. And we as humans and our instruments — AI audit systems — cannot parse and make transparent the nature of every black-box AI. Some hallucinations, unlike the extra-leggy spiders and erroneous autobiographies, will go unnoticed. Exaggeration in AI predictive finance models of stocks, in artificially produced code, in creditworthiness rankings, and in AI recidivism models. These are real-world domains with consequences for real individuals’ lives though. All of these manifestations of exaggeration, exacerbated by AI’s ubiquitous influence on the human world, therefore, has the potential to have an outsized impact on multiple spheres of society.
So, yes, it is important that we debate the ethics of academic and professional GPT use. AI images are indeed a pressing concern for the future of the Artworld. And the moral and legal personhood of AGI has never been more urgent. But let’s not blink and miss the devil of bias lurking in the technological details.