by Ali Minai
Among all the fascinating mythical creatures that populate the folklore of various cultures, one that stands out is the golem – an artificial, half-formed human-like creature that comes to us from Jewish folklore. Though the idea goes back much further, the most famous golem is the one said to have been created and brought to life by the great Rabbi Loew of Prague in the 16th century. Stories of various other golems have also come down through history, each with its own peculiarities. Most of them, however, share some common themes. First, the golem is created to perform some specific function. For example, the golem of Prague was created to protect the Jews of the city from pogroms. Second, the golem is capable of purposive behavior, but in a limited way. And third, the golem is activated by a human master by tagging it with a word (or words), and can be deactivated by removing or changing the word.
The golem has made many appearances in literature – especially in science fiction and fantasy – and its linkage with artificial intelligence is implicit in many of these instances. Though the golem is often portrayed as not particularly intelligent, the analogy with AI is clear in that the golem can act autonomously. More pertinent here, though, is another aspect of the golem’s behavior: Obedience. The golem has no purposes of its own, and is obedient to the desires of its master – though, interestingly, golems do rebel in some stories, which makes the analogy with AI even more apt.
The End of AI
The present time is seen by many as the golden age of AI, despite the fact that other “golden ages” have come and gone up in smoke in the past. The thinking is that this time AI has truly arrived because it is more grounded and has infiltrated down to the very core of modern human life. No aspect of life today – from medicine and finance to education and entertainment – remains unaffected in some fundamental way by AI. It is built into cars, cameras, and refrigerators. It analyzes investment portfolios and consumer preferences. It recognizes faces for security and for logging into digital devices. It lies at the core of Amazon, Google, Facebook, Apple, and many of the other technology and consumer giants that shape our lives. In these last fourteen months of the COVID pandemic, tools enabled by AI have been used extensively in data analysis, clinical practice, and pharmaceutical innovation. Indeed, so pervasive has AI become that, in many cases, it is not even noticed. We take it for granted that things in our lives – phones, watches, cameras, cars, vacuum cleaners, refrigerators, and even homes – will be “smart”. Increasingly, we expect things to understand what we are saying, and in the near future, we may expect them to understand our thoughts as well. The time is also at hand when more and more of the complicated things we do, from driving to writing legal briefs, will be left to AI. In a real sense, we humans are outsourcing our minds and bodies to algorithms. No wonder some very smart people are worried that AI may make slaves of us all, while others seem to be relishing the prospect of merging with smarter machines to become gods.
All this – and much more – is true. But skeptics have begun to push back on the euphoria. It is being noted that virtually all of AI’s success today is built upon machine learning algorithms that use extremely powerful computers and extremely large amounts of data to find patterns, and to exploit these patterns to very specific, narrowly defined ends, such as keeping a car safely on the road, summarizing a long document, or winning a game of Go. The discovery of pattern may be explicit or implicit, but invariably boils down to extracting statistical information from data. In the past, statistical analysis at this scale was limited by the complexity of the task and the lack of mathematical and computational tools. The triumph of modern machine learning lies in developing increasingly sophisticated, efficient, and data-driven computational methods for doing such analysis. “But is this AI?” ask the skeptics. It is too narrow, too specialized, too dependent on data and statistics. Most importantly, it is not grounded in experience, as natural intelligence is, so the machine has no real understanding, and can be fooled in laughable ways (I wrote about this topic in 3QD in 2019).
Another valid critique is that most successful AI today uses supervised learning, where the data for training machines has been pre-labeled by humans to indicate its meaning. What the machine does is to infer the rules by which those meanings – or labels – are related to the features of a given piece of data. What, for example, makes a film more popular among young people? Or what is the right move to make from a specific board position in chess? Humans answer such questions by using their intelligence, and to the extent that machines can do the same, this is seen as a hallmark of intelligence. But humans learn most things without explicit (supervised) instruction, learn from just a few examples, and can apply their intelligence to a vast range of tasks and problems – not just a narrow application for which they are trained. This type of broad, flexible, versatile natural intelligence is called general intelligence. The critics to today’s narrow AI often imply that true AI will only be achieved when machines come to possess artificial general intelligence, or AGI – and some skeptics are certain that this will never happen.
When John McCarthy and his colleagues met at Dartmouth College in 1956 to formulate the discipline of AI, these issues were not completely clear, but it was obvious that the ultimate goal was what today would be termed AGI. This is also the dream that has kept attracting some of the brightest minds in the world to the field of AI for decades, and has caught the fancy of SciFi enthusiasts and the general public. But critics say that this dream has been subverted by the lure of quick rewards that accrue from building superficially intelligent tools targeted at specific – often lucrative – applications, or by vacuous promotional exercises such as building machines that can play complicated games. It’s hard to argue with the validity of the critique, and even some of the stalwarts of the New AI are beginning to recognize that. But all the discussion of AI and AGI confounds two distinct – albeit related – issues: Versatility and autonomy. And this goes to one of the central questions of AI: What kind of thing do we want AI to be?
In practice, it is assumed implicitly that the purpose of AI is to improve human capacity by providing us with smarter tools – perhaps even to the point of implanting such tools into our bodies and turning ourselves into cyborgs. What AI technology seeks to build today is ever smarter servants for humankind: impersonal assistants and analysts with no goals, motivations, or preferences of their own. Or, as Amazon CEO Jeff Bezos has said, systems that provide an “enabling layer” to improve business.
There is now much talk of autonomy in the field of AI – especially with regard to vehicles and robots – but a deeper look at the desired autonomy shows that it is, in fact, closer to “obedience”. Consider Isaac Asimov’s so-called three laws of robotics, formulated for autonomous intelligent robots in a fictional context but an accurate reflection of the ethos of AI today:
- A robot may not injure a human being or, through inaction, allow a human being to come to harm.
- A robot must obey orders given it by human beings except where such orders would conflict with the First Law.
- A robot must protect its own existence as long as such protection does not conflict with the First or Second Law.
It is clear who is the master here and who the servant.
Even when autonomy is considered as desirable – as in the case of self-driving cars – the idea is not to add choice to the machine, but to obtain more obedient, unquestioning behavior by removing the risk of human fallibility from the machine. The ideal autonomous car would always bring its owner safely and exactly to their desired destination – no questions asked, and certainly with no attitude. A car with a mind of its own would be extremely unwelcome. So inbuilt is this assumption that most people don’t even see it. Of course, they assume, the entire purpose of engineering is to build instruments that work as their users demand.
Levels of Autonomy
To address this issue systematically, it is useful to look more closely at the concept of autonomy, defining it pragmatically as the ability to make decisions without external forcing. One can then identify different levels or types of autonomy in several ways. One of these is to consider the following four levels:
Level 0 – Passive Autonomy: This minimal type of autonomy is the ability of a system to choose one response out of many given a particular input, and to output it as information. Most people would not consider this autonomy at all, but it serves as a baseline from which the other levels can be distinguished. It is termed passive because the machine only generates information for human consumption and does not directly affect other systems. A face-recognition system identifying a person is an example. A more interesting example is the text generating system GPT-3, which produces responses of approximately human complexity with somewhat more than minimal autonomy but only in the domain of language. A large proportion of AI work today occurs at this level.
Level 1 – Active Autonomy: In this case, the intelligent system shows autonomous behavior by selecting specific actions in real-time in physical or virtual space. It may be argued that this is not fundamentally different from passive autonomy since action too is a response, but, as in the human context, it is useful to distinguish between “freedom of speech” (passive autonomy) and “freedom of action” (active autonomy) because the latter acts directly on the world. An example of active autonomy is a self-driving car that makes the decisions to stop, speed up, turn, etc., on its own while following a prescribed route. There is a significant amount of work currently underway on this type of autonomy as well.
Level 2 – Tactical Autonomy: Here, the system autonomously configures a plan, i.e., an extended course of action in order to accomplish a specific mission. The actions are not anticipated or prescribed. The machine is only given the goal by its human users and, with its repertoire of possible behaviors, must come up with its own plan. An example of this is an armed drone that is sent into enemy territory with the goal of detecting and destroying as many targets as possible while evading fire. Another example is a team of robots sent to another planet to set up a habitat for humans. This kind of autonomous AI is at or just beyond the cutting edge of research today, with examples confined mostly to simulations, demos, and special cases such as game-playing. More importantly, there is great reluctance to deploy AI with such autonomy in practice because it means significant loss of human control (though exceptions are now emerging.)
Level 3 – Strategic Autonomy: This is the highest level of autonomy where the intelligent system decides autonomously what goals or purposes it should pursue in the world. An AI system at this level would be a fully independent, sentient, autonomous being like a free human. Such AI is still strictly the stuff of science fiction and futurist literature. It is what prominent AI alarmists such as Stephen Hawking, Elon Musk, and Bill Gates have been warning about, but is also precisely the AI that the founders and visionaries of the AI project have always had in mind.
Currently, Level 0 dominates AI, with a significant amount of work around Level 1. The question is whether AI should stop there, move carefully up to Level 2, or seek to go all the way to Level 3.
There are several genuine conundrums here – not least the issue of responsibility: Who bears responsibility for the actions of a tactically or strategically autonomous system? There is also the question of values: Where will an autonomous AI system get its values, and will they be consistent with human values? A particularly germane and vexing place where this issue arises is with autonomous weapons: Should a drone have the autonomy to choose its own bombing targets in a battle, or even to decide which side it wants to fight on? The answer implicit in the current practice of AI is an emphatic “no!” A much larger, albeit more long-term, problem is that, if AGI does arrive, it will represent not just a new species but a totally new kind of life-form – the first non-Carbon based sentient life on Earth – unfettered by the constraints of biology and slow biological evolution. As many have suggested, this could pose an existential risk to humanity.
A Phase Space for AI
To explore this issue more deeply, it is useful to rethink the debate about AI in terms of the two attributes identified earlier in this article: Versatility and autonomy. Critics of the new AI have focused mainly on the former, complaining that AI systems are too narrowly focused, but the issue of autonomy is equally important and perhaps even more central to the future of AI. Using these two attributes, we can define for AI what physicists call a phase space – a space whose axes represent distinct attributes of systems within a specific domain. Every system within the domain then corresponds to a point in that space based on the degree to which it possesses each attribute (AI practitioners would call this a feature space, though there is a subtle difference that need not concern us here.) Figure 1 below shows this proposed phase space for AI. It should be noted that a comprehensive phase space for AI would include other dimensions too, such as style of learning, generativity, development, etc., but that is not relevant here.
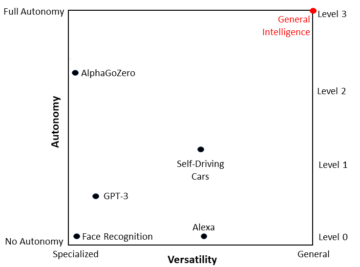
In the figure, autonomy is seen as a continuum rather than four discrete levels as described earlier, which are more useful for descriptive purposes. One can easily imagine AI systems that fall between the levels, such as s self-driving car that can autonomously change its path based on traffic congestion or its need for recharging. Similarly, versatility is seen as a continuum from highly-specialized systems to very general ones.
We are now in a position to address several fundamental issues. For example, various existing AI systems can be placed in the phase space, as shown in Figure 1. A face-recognition system is highly specialized and is autonomous only in a minimal sense, so it is close to the lower left corner. In contrast, a system like AlphaGo – DeepMind’s Go-playing system that beat one of the game’s grand masters – is highly specialized but basically at Level 2 in autonomy: It does its own planning – often surprising human experts – though it cannot change its goal of winning Go games. A subsequent system, AlphGoZero, is even more autonomous in its learning process, and learns without supervision. It is conceivable to have a specialized system that could be even more autonomous – choosing its own goals within a arrow domain. One can also imagine systems that are capable of a large number of different tasks, but have very little autonomy. Siri and Alexa can be considered partial approximations.
Back to AGI
So where does AGI fit into all this? A system that can do only one thing, no matter how autonomously, clearly does not have general intelligence. The case of an extremely versatile system with only passive autonomy is more debatable, and gets to the core of the AI project. This is not the place for an extensive discussion of the issues involved, but the essence of the debate is about the relationship between the brain and the body and their role in intelligence. The traditional view, rooted in theories of control and computation, sees the body as a purely mechanical system that is controlled directly by a central intelligence resident in the brain. In this formulation, one can discard the body and the brain would still be just as intelligent, though powerless to act out its desires. More importantly, intelligence is seen purely as a function of the brain, and thus, fundamentally as information processing in a computational system. The other view – termed embodied intelligence – holds that intelligence emerges from the interaction of the brain, the body, and a specific environment. Indeed, from this viewpoint, “brain” and “body” are not separate systems, but a single integrated physical system embedded in its environment. There are many nuances in the way this view has been framed, but the key point is that it sees intelligence as the dynamics of a physical system.
We are currently at an interesting stage in the history of AI where much of the conceptual vocabulary, the terminology, and even the practice of AI are all still rooted in the computational view, but both experimental evidence from biology and progress in engineering are inexorably building a case for the embodied dynamical view. From this viewpoint, intelligence is a characteristic of behavior, and thought is only a special case of that. A system with full AGI would not only be able think complicated thoughts but also behave in complicated ways – in physical or virtual space. Most importantly, it would learn through active exploration of its environment driven by its internal motivations, not by pure contemplation – and certainly not through off-line supervised learning like most AI systems today. In fact, it would not even have a framework in which to contemplate or learn if it was not experiencing the world through action. The more intelligent an embodied system is to be, the more autonomy it requires to enrich its experience, much as humans must grow from a low-autonomy infant into stages of greater and greater autonomy to achieve their full potential for intelligence. Indeed, from an embodied perspective, one might say that intelligence without autonomy is just a simulation of intelligence: Truly general intelligence can only be achieved by systems that have been let loose in the world. Thus, general intelligence lies at the upper right corner of the phase space – fully versatile and fully autonomous – and that is where AGI too will be found, no matter what path technology takes to get there.
Golem or God?
In his recent book Human Compatible, the prominent AI researcher Stuart Russell argues that AI should aim explicitly at being beneficial to humans rather than ever more intelligent and autonomous. The core tenet of this “human-compatible intelligence” proposal is that AI should have only as much versatility and autonomy as necessary to make it a useful servant to humans, and no more. Implicit in this the idea that AI must not develop to full AGI or anything close to it. Golems are acceptable, but anything more poses the risk of becoming a god.
Figure 2: Two scenarios for Safe and Risky AI.
But where in the versatility-autonomy phase space is the boundary between human-compatible AI and AI that might escape human control and enslave us like Skynet or Ultron – between golem and god? Figure 2 shows two possibilities for dividing up the phase space into “safe” and “risky” regions. In each case, the upper right region of the phase space is seen as risky. Scenario A on the left represents a more precautionary – some might say paranoid – case, preferring to stay well away from the AGI corner and severely limiting autonomy for all but the most specialized systems. Scenario B on the right is more adventurous, allowing a high level of autonomy in all but the most versatile cases. Much more sophisticated “human-compatible” servants – golems – will be built in both scenarios, though the scope for further growth is more limited in scenario A. The big question is whether humanity will cross the boundary into the red zone, where the growth of AI into superintelligence may become unstoppable. Those who discount this possibility make one of three broad arguments that can be summarized as: 1) AGI will never happen; and 2) AGI will not be interested in growing itself into a super-intelligence. Recently, Jeff Hawkins, in his book A Thousand Brains, has made an interesting third argument that AGI can be built safely by explicitly excluding from it those emotional elements that lead humans to lust for power. But is the boundary between safe and risky AI a real thing? And will we know when we are in danger of crossing it? No one can truly say, and we probably won’t face this prospect for a long time yet, but as the saying goes, it’s always too early until it’s too late.
Two primal human forces will shape the future of AI. One is the imperative of control that lies at the core of the modern ethos. The other is the irresistible urge to do things just because they are possible. There is little doubt that the algorithms of machine learning will keep improving, bringing more and more of what today requires human intelligence into their purview. But, on the present trajectory, the goals and purposes towards which these systems work will come from humans. Or at least that’s the plan, as it was for the creators of the various golems throughout history. It’s instructive to note that many golems – including the ones created by Rabbi Loew and Dr. Frankenstein – went rogue. Ultimately, they were all stopped because they were too stupid or too gullible, but AI is a much more subtle golem!
There was a time – back in the dark ages of the so-called symbolic AI – when it was assumed that professors were smarter than Nature, and that they (and their graduate students) could replicate the functions of intelligence as computer programs, building an AI that was wholly understood and thus controllable. That dream died long ago. The new vision is that AI can only be achieved by mimicking the structures and processes of the brain – by building ever larger and more complicated neural networks that learn from more and more data to perform functions identified with intelligence. This approach, which has been very successful in narrow AI, obtains its power at the cost of transparency. The huge neural networks of today’s deep learning can do a lot, but it is extraordinarily difficult to determine post facto how they are doing it. In fact, this has given rise to an entire science of explainable AI – as though actual human intelligence is ever truly able to explain itself! But, for all its power, the brain-inspired approach to AI is not that different philosophically from the old symbolic one. The main difference is that the transparent programs written top-down by smart humans have been replaced by opaque neural networks trained bottom-up on a lot of data. The final result in both cases is a computational artifact that purports to replicate a function of natural intelligence. Both are focused narrowly on a specific function, and can only produce more versatile intelligence through the combination of many interacting programs or many neural networks. With programs, this may, in principle, have led to a system that is still fairly transparent and controllable, but combinations of very complex, opaque neural networks are a different matter. But it goes further. To achieve any kind of non-rudimentary intelligence, the interacting neural networks must be embedded in an embodied system endowed with self-motivation, the autonomy to explore its world, and the ability to learn without supervision. We are now in the world of complex systems, where the combinatorial effects of multiple nonlinear systems can produce extremely unpredictable emergent behavior – just as happens with real intelligence. Such a machine does not learn from human-curated datasets, but seek its own experiences. It will not keep its motivation confined to things we approve of, or come to conclusions we prefer. Combinatoriality will create unexpected behavior, and learning will consolidate some of it. Before we know it, we will have at least a self-willed pet on our hands, if not a rebellious servant or a potential god.
Many have noted that AI is fundamentally different from every other artifact built by human engineering because it can autonomously improve its own capabilities. Those arguing for a safe AI assume that this self-improvement can be tracked and controlled, but – as the argument above asserts – that is not possible because of the fundamentally complex and emergent nature of intelligence. It is impossible to know where the boundary between safe and risky AI truly is, or to prevent machines from crossing it. AI is an all-or-none enterprise: Either we make systems that can be meaningfully intelligent, i.e., embodied, self-motivated, and self-learning, or we just keep building slightly smarter screwdrivers at low levels of autonomy. But, in fact, that choice has already been made; we are already on the slippery slope, and there are consequences.
As the philosopher Mark Taylor says in his recent book, Intervolution: Smart Bodies, Smart Things, “The machines we have programmed to program themselves end up programming us.” Long before there is a danger of machines becoming gods, they will become essential to our lives. We already see that with non-intelligent machines: It is almost impossible to live in the world today without access to motorized transportation or instant communication. What dependencies lie in store for us with AI? We will help our machines get smarter because we will need them more and more as our servants. Long before they become their own masters, we will already be their slaves. Ultron can wait.