by Richard Passov
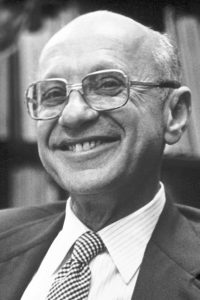
Milton Friedman, in his essay The Methodology of Positive Economics[1], first published in 1953, often reprinted, by arguing against burdening models with the need for realistic assumptions helped lay the foundation for mathematical economics. The virtue of a model, the essay argues, is a function of how much of reality it can ignore and still be predictive:
The reason is simple. A [model] is important if it explains much by little, that is, if it abstracts the common and crucial elements from the mass of complex and detailed circumstances surrounding the phenomena to be explained and permits valid predictions on the basis of them alone.
Agreement on how to allow predictive models into the canon of Economics, Friedman believed, would allow Positive Economics to become “… an Objective science, in precisely the same sense as any of the physical sciences.” What Friedman coveted can be found in a footnote:
The … prestige … of physical scientists … derives … from the success of their predictions … When economics seemed to provide such evidence of its worth, in Great Britain in the first half of the nineteenth century, the prestige … of … economics rivaled the physical sciences.
Friedman appreciated the implications of the subject as the investigator, to a degree. “Of course,” he wrote, “the fact that economics deals with the interrelations of human beings, and that the investigator is himself part of the subject matter being investigated…raises special difficulties…
But he loses the value of his observation to a spate of intellectual showboating:
The interaction between the observer and the process observed … [in] the social sciences … has a more subtle counterpart in the indeterminacy principle … And both have a counterpart in pure logic in Gödel’s theorem, asserting the impossibility of a comprehensive self-contained logic …
The absence of an ability to conduct controlled experiments, according to Friedman, was not a burden holding back progress or unique to the social sciences. “No experiment can be completely controlled,” he wrote and offered astronomers as an example of scientists denied the opportunity of controlled experiments while still enjoying the prestige he coveted.
But though moving economics forward as a positive science – one where predictions are formulated through math and then tested against alternative formulations – he did not want to see mathematics supplant economics. “Economic theory,” he wrote, “must be more than a structure of tautologies … if it is to be something different from disguised mathematics.”
When Friedman penned his article, the simplest mathematical formulations exhausted computational capacity.
* * * * * *
Tom Griffiths, Professor of Psychology and Cognitive Science at Princeton, in a recently published essay [2,3,4],writes:
We’re trying to understand why people do what they do and what the cognitive processes are that underlie the data we find in the world that are a consequence of human behavior.
His method of meeting this challenge is to turn it “… into a math problem … the kind of thing that we can imagine getting a computer to solve.” As these problems are mathematized, tools from AI, statistics and machine learning will serve as the basis for “… hypotheses about how human cognition might work.”
Armed with these hypotheses according to Griffiths, “… we can run experiments that test predictions that come out of those models, and then we can use that as a tool for digging deeper into how human cognition works and how people solve those kinds of problems.
* * * * * *
To frame economics within mathematics, equal in importance to ignoring assumptions is the need to follow logic, which in turn imposes the need for rational decision agents. In an earlier work [5], Friedman argued that “…consumer units…” behave as if they were rational decision agents. On this point, Griffiths writes:
…following the rules of logic and probability was assumed to be the essence of rational thinking … Without the principles of rationality, there is little guidance for how to translate assumptions about cognitive processes into predictions about behavior and how to generalize from our data.
Kahneman and Tversky published Prospect Theory in 1979. That, along with their efforts to establish the field of Behavioral Finance, seemingly undercut the foundations of an economics based on humans as rational (logical) decision agents. But according to Griffiths when viewed against the constraints of a hypothetical, yet feasible, construct of cognition Kahneman and Tversky’s behavioral anomalies become rational decision-making tools.
Economists have also wrestled with bounded rationality [6], and heuristics – Fast and Frugal short cuts arising from the need to make critical decisions under time, and therefore computational, constraints. [7]
Those economists view these strategies as outcomes of an ecological process – that is, a product of a mechanism driving change along with a process that favors outcomes that add a survival advantage. (In mathematical terms, an ecological process can be interpreted as confining a future state to one that is likely to arise from the current state, which in turn contains all relevant information from prior states – aka a Markov Process.)
* * * * * *
Griffiths operates in a world that Friedman failed to imagine – a data-rich world “cast up” in part, as Friedman noted, “by the experiments that happen to occur.” But today data is also the output of experiments designed to produce results that are tested against empirical data.
According to Griffiths, the test is of the predictive accuracy of a “function-first approach” that begins by postulating “… an abstract computational architecture, that is a set of elementary operations and their costs, with which the mind might solve [the] problem.” Then, based on a cost of computation* the optimal algorithm for solving the problem is identified.
“This enterprise,” Griffiths writes, “is important for a couple of reasons.” First, “data … [is] becoming an increasingly important part of our lives” and; “…having good models of how people think and behave is relevant to helping AI systems better understand what people want.”
What are these systems trying to understand?
How [to] make recommendations to somebody? How [to]… identify people who other people will want to be friends with? How [to] … figure out, based on their actions, what people are interested in? … How [to] figure out what kinds of things [people] will apply a tag to, what kinds of images they’ll label in a particular way?
But how can mathematizing a decision problem so that it can be solved as a constrained optimization exercise on a “… abstract computational architecture …” help?
… If you’ve got a computer that has information about the structure of the problem that you’re solving and can communicate that information back to you …, then we can build a system that will help guide people to make better decisions.
For example, Griffiths writes, we can gamify a problem, dropping little rewards along the way, guiding you, me, us to better decisions.
* * * * * *
Of course this is happening already. According to Griffiths, “In the technology industries right now, there’s a lot of data on human behavior.” This data is being used to “… make inferences about your preferences and desires, and then figure out how [to] best satisfy those (and take some of your dollars in the process).”
But if, as some believe, our algorithms for decision making are the result of an ecological process, what are the implications of better understanding “… how you can use the computer to change the environment that the human being is in so that that human being ends up making better decisions …”
Can the process that arrived at our behaviors change, say across a generation or even over an individual’s life span? If this is the case, and there’s reason to believe it is [8], then Griffiths is correct when he writes:
… we’re at a moment where there’s a unique opportunity for … cognitive science to have a broader impact.
If our world is gamified and if we’re programed to seek the pleasure of winning, then will the process that developed fast and furious heuristics evolve behaviors that mimic the resource-rational algorithms used to model our behaviors?
If so, if algorithms that model human decision-making processes create processes that output desired data, or as Griffiths says, output our likes, then it stands to reasons that machines are not destined to think like us. We are destined to think like machines.
* * * * * *
*cost of computation: According to Griffiths, resource-rational anchoring-and-adjustment, for a certain set of problems, in part, reduces to the following algorithm
tı = arg min [E Q(ˆXt) [cost(x, ˆxt) – d · t]],
where tı is the optimal number of guesses a person should make before deciding an answer. Notwithstanding first impressions, a decent first year math student wouldn’t run from this, though she might find one aspect curious.
According to Griffiths,ˆxt is one in a series of guesses that our human makes and cost (x, ˆxt) is the cost of this estimate, where x is the correct decision. So to implement this algorithm, one must have some way of knowing the cost associated with a wrong estimate which in turn requires knowing the right answer. Which reminds me of what an ex-spouse once said after I worked through the night in search of an answer: “Why didn’t you just guess. Then you wouldn’t have been late for dinner.”
The above is not a criticism of Griffiths’ model. Though I reserve the right to doubt that humans make decisions in the manner that Griffiths suggests, I have no reason to doubt the efficacy of his model. As Friedman wrote, it’s not necessary that the plant manager actually calculate marginal costs before deciding output levels. It’s only necessary that it seems as though that were the case.
Yet there is something to be warned against in this formulation. If I were to arrive at a decision by following Griffith’s algorithm, then it is I who weighs the cost of being right or wrong. But if I am in an environment controlled by a computer rewarding me toward the right answer, then who weighs the costs?
* * * * * *
[1]Milton Friedman, Essays in Positive Economics. In, The Methodology of Positive Economics, pp. 3–43, (1953).
[2]Tom Griffiths, Aerodynamics of Cognition, A Conversation with Tom Griffiths. Edge.org. August 21st, 2018.
[3]F. Lieder, T. Griffiths, Q. Huys and N. Goodman, The Anchoring Bias Reflects Rational Use of Cognitive Resources. Psychonomic Bulleting & Review, May 2017.
[4]B. Christian, T. Griffiths, Algorithms to Live By: The Computer Science of Human Decisions. Henry Holt and Company, New York (2016).
[5]M. Friedman; L. J. Savage, The Utility Analysis of Choices Involving Risk. The Journal of Political Economy, Vol. 56, No. 4. August, 1948, pp. 279-304.
[6]Herbert Simon, A Behavioral Model of Rational Choice, in Models of Man, Social and Rational: Mathematical Essays on Rational Human Behavior in a Social Setting. New York, Wiley (1957).
[7]D. Goldstein, G. Gigerenzer, Models of Ecological Rationality, Psychological Review, 2002, Vol. 109, No 1, 75 –90.
[8]Maryanne Wolf, Proust and the Squid: The Story and Science of the Reading Brain, Thriplow: Icon Books, (2008).